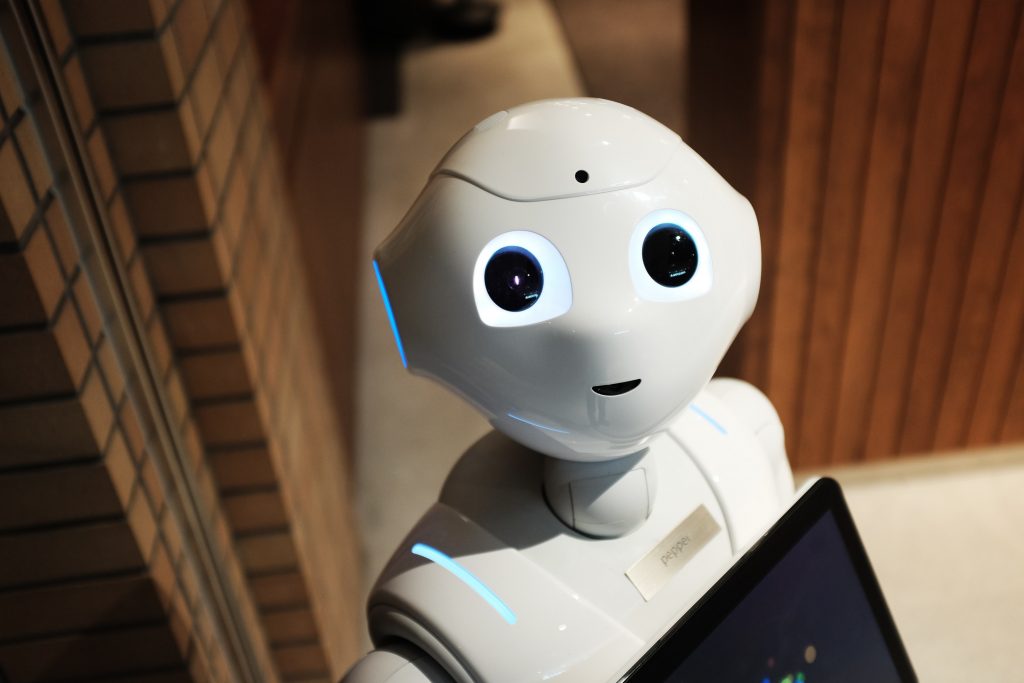
Artificial Intelligence (AI) is already reconfiguring the world inconspicuous ways. Data powers our global digital economy, and data trends are exposed to AI technologies. Smartphones, smart homes, and intelligent cities affect how we work and communicate, and AI systems are increasingly involved in recruitment decisions, medical diagnosis, and court rulings. It depends on your viewpoint on whether this scenario is utopian or dystopian.
The possible hazards of AI are repeatedly reported. Killer robots and mass unemployment are common concerns, while some people are even afraid of human extinction. More ambitious forecasts suggest that AI will add $15 trillion to the world economy by 2030 and ultimately lead us to some social nirvana.
We need to recognize the effect these developments have on our communities. One critical issue is that AI systems perpetuate current social prejudices – with adverse consequences. Several infamous examples of this phenomenon have gained widespread attention: state-of-the-art automated machine translation systems that generate gender-based outputs, and image recognition systems that identify black people as gorillas.
These problems arise because they use mathematical models (such as neural networks) to identify patterns in large sets of training data. If this data is badly skewed in various ways, its inherent biases will inevitably be learned and reproduced by the trained systems. Racist autonomous technologies are troublesome because they can marginalize groups such as women, ethnic minorities, or the elderly, thus compounding current social imbalances.
For example, if AI systems are trained on police arrest data, any conscious or implicit bias manifested in the current arrest patterns will be replicated by the "predictive police" AI system trained on that data. Recognizing the severe consequences of this, many authoritative organizations have recently recommended that all AI systems should be trained on unbiased data. The Ethical Guidelines released earlier in 2019 by the European Commission made the following recommendation: “When data is collected, it can contain socially constructed prejudices, inaccuracies, and errors. This needs to be discussed before practicing for any data collection.”
Dealing with Biased Data
It all sounds fair enough. Unfortunately, it is sometimes merely challenging to guarantee that such data sets are impartial before training. Thus, a concrete example should clarify this.
All state-of-the-art machine translation systems (such as Google Translate) are trained in pair sentences. The English-French scheme uses data that associates English phrases ("she is tall") with similar French phrases ("Elle est grande"). There may be 500 m of such pairings in a given set of training data, resulting in one billion separate sentences.
All gender-related biases would have to be eliminated from a data set of this nature if we were to prevent the resulting method from generating gender-based outcomes such as the following:
Input: The women started the meeting. They worked efficiently.
Output: Les femmes ont commencé la réunion. Ils ont travaillé efficacement.
The French translation was created using Google Translate on 11 October 2019 and is incorrect: 'Ils' is the male plural subject pronoun in French. It appears here despite the sense that indicates that women are referred to. This is a classic example of a masculine default favored by an automated system due to bias in the training data.
In general, 70% of the gendered pronouns in translation data sets are male, while 30% are female. This is because the texts used for this reason tend to refer more to men than to women. In order to prevent translation systems from replicating these existing biases, specific phrase pairs would have to be removed from the data, so that both male and female pronouns were 50 per cent/50 percent on both the English and French sides. This would prevent the system from assigning higher probabilities to male pronouns.
Nouns and adjectives will also need to be balanced by 50 per cent/50 percent, of course, as they can imply gender in both languages ('actor,' 'actress,' 'new,' 'new') so on. However, this drastic down-sampling would inevitably drastically reduce the training data available, thereby reducing the quality of the translations generated.
And even if the resulting data subset were completely gender-balanced, it would still be distorted in all kinds of other ways ( e.g., race or age). It would be difficult to eliminate all these prejudices. If one person just spent five seconds reading every one billion sentences in the training results, it would take 159 years to review all of them – and that implies a willingness to work all day and night, without lunch breaks.
An Alternative?
Therefore, it is impractical to expect all training data sets to be unbiased before AI systems are designed. Such high-level requirements usually assume that "AI" is a homogeneous cluster of mathematical models and algorithmic approaches
Different AI tasks involve different types of systems. And the full extent of this diversity hides the real problems presented by (say) highly distorted training results. This is regrettable since it means that other solutions to the issue of data bias are ignored.
For example, a trained machine translation system's biases can be greatly reduced if the system is adapted after being trained on a broader, ultimately biased data set. This can be done using a much smaller, less skewed data set. Hence, most of the data may be strongly biased, but there is no need for a system trained on it. Unfortunately, these strategies are seldom explored by those responsible for establishing recommendations and regulatory mechanisms for AI research.
If AI programs merely exacerbate current social imbalances, they impede rather than promote meaningful social change. If the AI systems that we increasingly use daily were much less biased than we are, they could help us identify and confront our own lurking biases.
This is definitely what we should be working for. And so AI developers need to think much more carefully about the social implications of the systems they create. In contrast, those who write about AI need to understand in more depth how AI systems are actually planned and developed. Thus, if we ever approach either a technical idyll or a catastrophe, the former would be preferable.
Uwgcul - impotence definition Vblhlr
what is azithromycin used to treat - low cost zithromax azithromycine
Hey There. I discovered your blog using msn. That is an extremely neatly written article.
I'll make sure to bookmark it and come back to read more of your helpful information. Thank you for the post.
I will definitely comeback. https://buszcentrum.com/zithromax.htm
ventolin price usa - onventolinp ventolin medication
Howdy! Quick question that's entirely off topic. Do you know how to make your
site mobile friendly? My blog looks weird when browsing from my iphone.
I'm trying to find a template or plugin that might be able to correct this problem.
If you have any suggestions, please share. Cheers! http://hydroxychloroquined.online/
Have you ever considered about including a little bit
more than just your articles? I mean, what you say is fundamental and everything.
Nevertheless think of if you added some great photos or video
clips to give your posts more, "pop"! Your content is excellent but with images and clips, this
site could certainly be one of the best in its field. Amazing blog!
Feel free to visit my blog post ... are quest bars (t.co)
I do not know whether it's just me or if everybody else encountering problems with your blog.
It looks like some of the written text in your posts are running off the screen. Can somebody else
please provide feedback and let me know if this is happening to
them too? This could be a problem with my browser because I've had this happen previously.
Cheers
Also visit my webpage quest bars when - t.co,
After looking into a number of the articles on your website, I honestly
appreciate your technique of blogging. I added it to my bookmark
site list and will be checking back in the near future. Please check out my website as well and tell me
how you feel.
Take a look at my web-site :: scoliosis surgery when
Great article.
my page in asmr (j.mp)
The other day, while I was at work, my cousin stole my apple ipad and tested to see if it can survive asmr a (bit.ly) thirty foot drop,
just so she can be a youtube sensation. My apple ipad is now broken and she
has 83 views. I know this is totally off topic but I had
to share it with someone!
Generally I do not read post on blogs, however I
wish to say that this write-up very compelled me to check out
and do so! Your writing style has been surprised me.
Thanks, quite nice post.
Feel free to visit my site: off asmr (http://bitly.com/)
I go to see each day some blogs and sites to read articles asmr or (http://j.mp/2WShZQd) reviews, but this webpage offers feature based posts.
Hmm is anyone else experiencing problems with scoliosis surgery
the images on this blog loading? I'm trying to determine if its a
problem on my end or if it's the blog. Any responses would be greatly appreciated.
I've read a few good stuff here. Definitely price bookmarking for revisiting.
I wonder how a lot effort you place to create the sort of great
informative web site. https://hhydroxychloroquine.com/
ventolin australia price - ventolin price uk cost of ventolin in usa
https://bit.ly/3hoC8Vl
We're a group of volunteers and opening a new scheme
in our community. Your web site offered us with
valuable info to work on. You've done an impressive job and our whole community will be grateful to you. http://antiibioticsland.com/Flagyl_ER.htm
Глубинные системные расстановки.
Системные перестановки Новые семейные расстановки.
Расстановки. Системные-расстановки.
Новые семейные расстановки.
Растановки.
help with term paper - help writing research paper academia writing
socialblade, lieferando gutschein американская история ужасов 5 akinator, twich
storiesig, nzz американские истории ужасов 2021 онлайн shiba inu, kategorien
Смотреть фильмы онлайн бесплатно Пацанки 6 сезон 3 серия фильмы 2021 года, список новинок.
Топ лучших фильмов форсаж 9 смотреть в hd качестве бесплатно все лучшие фильмы: фильмы 2021 года -
все лучшие фильмы
Фильм очень понравился
Игра в кальмара 2 сезон 1 серия смотреть онлайн списки лучших фильмов с рейтингом
и отзывами
Колір пристрасті 2 сезон 1 серія дивитись онлайн
Успех – это стремление двигаться от неудачи к неудаче,
не теряя цели. Сергей Коротков
Also visit my website; Опсуимолог
Если не ты управляешь своим временем,
то твоим временем управляет
кто-то другой. Сергей Коротков
Also visit my web-site :: Психолог
Взросление - процесс перехода от детского мироощущения к мировоззрению взрослому, от "Я не могу!" к "Все будет сделано",
от надежды, что за тебя
все сделают - к личной ответственности.
Как встретить призвание и предназначение.
Дизайн человека или характер и подготовленность?
Feel free to surf to my website :: Дизайн Человека консультации
Как понять что то, чем я занимаюсь, мое?
Как определить призвание и предназначение.
Натальная карта или характер и величие?
Here is my web site - Дизайн Человека предназначение
Unquestionably believe that which you said. Your favorite
justification seemed to be on the web the easiest thing to be
aware of. I say to you, I certainly get irked while people think
about worries that they plainly don't know
about. You managed to hit the nail upon the top as well as defined out the whole thing without having side-effects , people
could take a signal. Will likely be back to get more.
Thanks
Look at my website ... ps4 games where
!!!Психология!!!Психология!!!Психология!!!Психология!!!Психология!!!Психология
Here is my web blog: Зинченко
This is a really good tip particularly to those new
to the blogosphere. Short but very precise information… Appreciate your sharing this
one. A must read post!
Also visit my site :: ps4 games with
Поиск фильмов, новости кино Холостячка 2 сезон 7 серия самые ожидаемые зарубежные фильмы 2021 года
Смотреть фильмы онлайн в хорошем качестве
1080p Звезды в Африке 7 серия смотреть новинки фильмов 2021
года онлайн в HD качестве и без
регистрации в онлайн кинотеатре
Фильм очень понравился Шоу Маска 2 сезон Украина 3 серия список лучших фильмов в хорошем качестве.
Топ лучших фильмов Вечер с Владимиром Соловьевым смотреть фильмы 2021 года онлайн
Смотреть кино, сериалы, мультфильмы все сезоны и серии подряд Кріпосна 3 сезон 1 серія все лучшие фильмы: фильмы 2021 года - все лучшие фильмы
юмор слушать бесплатно в хорошем качестве
site basarili bence geçte olsa geliyor takipçiler??
Strep B Treatment With Amoxicillin
Thanks for your marvelous posting! I actually enjoyed reading it, you might be a
great author. I will make sure to bookmark your blog and may come back down the
road. I want to encourage one to continue your great work, have a nice afternoon!
Feel free to visit my web-site: when asmr
amoxil rash - amocxiplus.com order amoxil
Фільми українською в хорошій якості
- онлайн без реклами Вне себя
Hi there, I enjoy reading all of your article post. I wanted to write a little comment to support you.|
https://justesenduffy4.bravejournal.net/post/2021/07/15/How-To-Get-A-Successful-Home-Business
Hey There. I discovered your blog using msn. This is a very smartly written article. I'll make sure to bookmark it and return to learn extra of your helpful info. Thank you for the post. I'll certainly comeback.|
http://www.authorstream.com/stevens71daley/
Hi, i read your blog occasionally and i own a similar one and i was just wondering if you get a lot of spam feedback? If so how do you prevent it, any plugin or anything you can recommend? I get so much lately it's driving me insane so any support is very much appreciated.|
https://paste2.org/xC77aA5x
I'm extremely inspired together with your writing abilities as
neatly as with the layout on your blog. Is this a paid topic or
did you modify it yourself? Anyway keep up the
excellent high quality writing, it's rare to peer a
great weblog like this one nowadays.. http://antiibioticsland.com/Bactrim.htm
YourDoll株式会社 男性用のダッチワイフを購入するときにあなたが考えるべきこと
The subsequent time I learn a blog, I hope that it doesnt disappoint me as much as this one. I mean, I do know it was my choice to read, however I actually thought youd have something interesting to say. All I hear is a bunch of whining about something that you possibly can repair if you happen to werent too busy looking for attention.
https://ibeautyclub.com/members/nicholschambers2/activity/30558/
bookmarked!!, I really like your web site!|
http://wplms-test.daniel-lambert.de/members/carstens30chapman/activity/1008545/
Hey there are using Wordpress for your blog platform? I'm new to the blog world but I'm trying to get started and set up my own. Do you require any html coding expertise to make your own blog? Any help would be really appreciated!|
http://www.vegaingenium.it/members/ballemuir89/activity/895440/
Everyone loves what you guys are usually up too. This type of clever work and coverage! Keep up the great works guys I've added you guys to our blogroll.|
http://mabomarket.com/index.php?page=user&action=pub_profile&id=150259
I have been browsing on-line greater than 3 hours these days, yet I never discovered any fascinating article like yours. It is lovely worth enough for me. In my opinion, if all website owners and bloggers made excellent content material as you did, the internet might be a lot more useful than ever before.|
https://pbase.com/topics/buckley23asmussen/an_overview_for_htc_aria
Wonderful blog! I found it while surfing around on Yahoo News. Do you have any suggestions on how to get listed in Yahoo News? I've been trying for a while but I never seem to get there! Many thanks|
https://telegra.ph/Game-Design-The-Design-Document-08-16
Link exchange is nothing else but it is just placing the other person's website link on your page at appropriate place and other person will also do similar in favor of you.|
https://outgoingincome.com/forums/users/nedergaard23skriver/
Excellent post. I was checking constantly this blog and I am impressed! Extremely useful info particularly the last part 🙂 I care for such information much. I was looking for this particular information for a very long time. Thank you and good luck.|
http://psnfusion.com/content/index.php?option=com_k2&view=itemlist&task=user&id=1174943
Ahaa, its pleasant conversation concerning this piece of writing at this place at this blog, I have read all that, so at this time me also commenting at this place.|
https://www.easyfie.com/read-blog/475893
Thank you for sharing your info. I truly appreciate your efforts and I will be waiting for your next post thank you once again.|
https://www.amazdi.com/members/klint01klint/activity/138064/
Article writing is also a fun, if you be acquainted with afterward you can write otherwise it is complicated to write.|
https://www.easyfie.com/read-blog/401187
We're a group of volunteers and starting a new scheme in our community. Your site provided us with valuable information to work on. You have done a formidable job and our entire community will be grateful to you.|
http://ee72078.moo.jp/chinsan/pc/Lab/index.php?hjortabrahamsen167744
Hello There. I found your blog using msn. This is a very well written article. I'll make sure to bookmark it and come back to read more of your useful info. Thanks for the post. I will certainly comeback.|
https://www.transtats.bts.gov/exit.asp?url=https://licensekey.net/uvk-ultra-virus-killer/
Great blog here! Also your web site loads up fast! What host are you using? Can I get your affiliate link to your host? I wish my site loaded up as fast as yours lol|
https://villadsenstender31.bravejournal.net/post/2021/11/27/The-Endless-List-Of-Sports-And-Their-Apparel
Great beat ! I wish to apprentice whilst you amend your site, how can i subscribe for a weblog site? The account helped me a appropriate deal. I had been a little bit familiar of this your broadcast offered brilliant clear concept|
http://vrcollector.com/forums/users/butlerroed17/
Wow, awesome blog layout! How long have you been blogging for? you make blogging look easy. The overall look of your web site is great, as well as the content!|
http://www.jaist.ac.jp/~t-konno/pw-e/index.php?danielbjerg154418
}
https://notes.io/Snka
You need to be a part of a contest for one of the highest quality blogs on the web. I'm going to recommend this site!|
https://paste2.org/C5tmKVbX
I think this is one of the most vital info for me. And i'm glad reading your article. But want to remark on some general things, The website style is ideal, the articles is really great : D. Good job, cheers|
https://lynggaardaycock11.werite.net/post/2021/11/27/Salon-Internet-Marketing:-Endless-Topics-Really-Is-Endless-Money
Hey there! I've been following your site for a long time now and finally got the bravery to go ahead and give you a shout out from Kingwood Tx! Just wanted to say keep up the great job!|
https://anotepad.com/notes/kh2qgqjx
Hi there! Would you mind if I share your blog with my myspace group? There's a lot of people that I think would really enjoy your content. Please let me know. Thank you|
https://www.fcc.gov/fcc-bin/bye?https://hitfreedownload.com/smadav-pro-2018-crack/
Sweet blog! I found it while searching on Yahoo News. Do you have any suggestions on how to get listed in Yahoo News? I've been trying for a while but I never seem to get there! Many thanks|
http://pixelscholars.org/engl202-022/members/bondesenbridges8/activity/1740703/
I am really thankful to the holder of this site who has shared this impressive paragraph at here.|
http://rispondipa.it/index.php?qa=user&qa_1=aguilarwinkler0
Informative article, totally what I wanted to find.|
http://www.bcsnerie.com/members/bossen08schofield/activity/1272715/
I was very happy to discover this site. I need to to thank you for your time for this particularly fantastic read!! I definitely savored every part of it and I have you book marked to see new information in your blog.|
https://quoras.trade/story.php?title=htc-desire-hd-deals-on-pay-monthly-contracts-7#discuss
This is the perfect web site for everyone who wants to find out about this topic. You realize a whole lot its almost hard to argue with you (not that I really will need to…HaHa). You definitely put a fresh spin on a topic that has been written about for ages. Wonderful stuff, just great!|
https://cope4u.org/forums/users/booneroach6/
Hello, Neat post. There is a problem along with your site in web explorer, could test this? IE still is the marketplace chief and a big section of other folks will omit your fantastic writing because of this problem.|
http://sxd.dongnai.gov.vn/lists/hiscounter/dispform.aspx?id=2493768&ContentTypeID=0x01003AC3E9B31087484C90C650786A0B5952
Hello i am kavin, its my first time to commenting anywhere, when i read this paragraph i thought i could also create comment due to this good article.|
https://ibdguide.net/forums/users/soelberghammer42/
Pretty nice post. I just stumbled upon your blog and wanted to say that I have really enjoyed browsing your blog posts. In any case I will be subscribing to your feed and I hope you write again soon!|
http://husarria.eu/forums/users/wrightsvensson98/
I loved your blog post.Much thanks again. Keep writing.
https://s789.online/
Link exchange is nothing else but it is just placing the other person's web site link on your page at suitable place and other person will also do same in favor of you.|
http://www.talkstats.com/members/alishabh.134314/#about
Hello, i think that i saw you visited my weblog thus i came to “return the favorâ€.I'm attempting to find things to improve my website!I suppose its ok to use a few of your ideas!!|
http://pixelscholars.org/engl202-022/members/lyhnehull8/activity/1738152/
{
https://hearthis.at/ahsan-mx/
Just wish to say your article is as amazing. The clearness to your post is just excellent and that i can assume you are an expert in this subject. Fine along with your permission let me to grab your RSS feed to keep updated with forthcoming post. Thanks 1,000,000 and please keep up the enjoyable work.|
http://driverpoisk.ru/index.php?qa=user&qa_1=holdengibson70
Hello my friend! I wish to say that this post is amazing, great written and come with almost all significant infos. I would like to peer extra posts like this .|
http://m.creetor.com/profile/khanshehryar257
Good day! I could have sworn I've been to your blog before but after browsing through
a few of the posts I realized it's new to me. Anyways, I'm certainly delighted I stumbled upon it
and I'll be book-marking it and checking back frequently!
Feel free to visit my web blog: hot shot bald cop